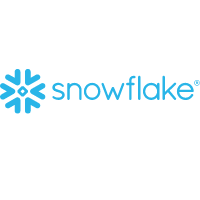
Snowflake
View Brand PublisherExperts weigh in on bringing scale and agility to complex data landscape
At a recent panel discussion, experts discussed the costs and consequences of leveraging LLM models, the benefits of various data architectures, and how companies can bring scale and agility to their data models.
Data plays a pivotal role in today's digital era, influencing the success of businesses worldwide. However, companies often find themselves in a fix on account of the volume of data, presence of multiple data sources in different locations, and varied quality of data. Hesitancy in changing data strategies and leveraging new technologies make it tough to boost efficiency and speed, integrate diverse data sources, and extract valuable insights in the long term.
This begs a question: can companies craft comprehensive and agile data strategies to create a lasting impact? Snowflake, in association with YourStory, brought together experts for a discussion on ‘Build to Scale: Integrating Data, AI, and Applications for Long-Term Success’.
The roundtable featured thought leaders and experts from a range of industries: Mrinal Rai, Co-founder and CPO, Intugine Technologies; Rohit Nambiar, VP of Engineering, CloudSEK; Venkata Rao, Head of Engineering, Needl.ai; Prakash Jothiramalingam, VP - Engineering, Lead Squared; Pramod Agarwal, CTO, IBSFINtech; Pradeep Sreeram, Head of Engineering, Gnani.ai; and Rajat Dwivedi, Director - Engineering, Plivo.
The panel began with a short keynote address by Pravin Fernandes, Head, Commercial Business - Snowflake, India, and was moderated by Shivani Muthanna, Director - Strategic Partnerships & Content, YourStory Media.
The challenges of big data
Businesses and organisations now collect data from a range of sources, but it remains to be seen if it is being used effectively. The panellists shared challenges around using data effectively.
Mrinal Rai said Intugine Technologies had to manage many integrations to ensure that it was able to give clients 100% visibility. It also faced significant data sanitisation challenges.
“We have an infinite number of sources from which data comes. Our goal is to bring data on to a single platform, show it to our client in simple language so he knows where his shipment is, or if there are any delays/halts that can result in missing out on sales or deliveries. That is something we are trying to figure out,” he said.
Cloud SEK, a company that leverages AI to predict and prevent cyber threats, deals with an influx of data from all over the world. Nambiar shared how this data was necessary to help clients safeguard operations from cyber-attacks. He spoke about the need to discover new patterns in data to stay ahead of the curve and serve customers better.
Pramod Agarwal, of IBSFINtech, said that while data scaling wasn’t a problem, data variability was a challenge. He spoke about a variety of data structures generated by different financial instruments, citing unstructured data as the biggest challenge for the organisation.
Balancing the benefits of AI with its cost
The need for small language models (SLMs) was discussed at length. While large language models (LLMs) have made exciting advances in the field of AI, their size necessitates significant computing resources to operate, boosting the cost of operations.
Panellists discussed strategies to reduce the cost of running these models. They agreed that SLMs were ideal in a specific domain context. However, given the wide range of AI applications across industries, they agreed that a one-size-fits-all solution would prove ineffective. Most panellists recommended that companies balance factors like speed to market with the cost of AI.
Rajat Dwivedi, Director - Engineering, Plivo, spoke at length about his experience with LLMs. He shared that the company initially contemplated leveraging LLMs to follow others in the field. However, the leadership at Plivo recognised that this approach was incorrect.
He urged companies to find their problem statements first and then decide if an LLM, a dataset, or a retrieval augmented generation (RAG) pipeline could resolve it effectively instead of “choosing a solution and finding an issue for it to solve”.
Pradeep Sreeram at Gnani.ai shared this thoughts on LLMs, saying “On the one hand, every time a model comes out, we wonder when it will take over our jobs. That is how intelligent it is becoming. On the other hand, I also believe that these models will become truly scalable when they can run on a lower hardware, which will be really cost effective” he said.
Enhancing data protection and security
There is a growing need for enhanced security measures as enterprises continue to create and store vast amounts of data for analysis.
For Rohit Nambiar, VP of Engineering, CloudSEK, ensuring data privacy and security continues to be a complex endeavour. Companies must navigate various national regulations, while contending with international compliances. In India, financial entities are required to keep certain types of data, which requires overhauling data practices and staying agile in the face of ever-evolving governance laws.
Cloud SEK is building its own language model, but remains cautious about utilising the data, given the conflicting regulations in different regions. Nambiar also said that Cloud SEK runs the data through a stringent process for clients who opt for cloud over on-premises security. The company identifies the data, where it is taken from, where it will be stored, and who should have access.
Best practices for AI integration
Praveen Fernandes, of Snowflake, shared that data integration strategies should be unique to each and every organisation.
“We have seen people who leverage multiple data strategies, are on their own data journeys, or at a different stage of data maturity. Organisations know their businesses and are capable of identifying what strategy will work best,” he said.
Fernandes stressed that companies should be free to choose between a decentralised data mesh architecture or a data vault. This choice also comes down to the teams required to manage these architectures. For instance, opting for a decentralised approach like a data mesh means setting up a lean central team and having analysts and AI experts at every business unit, he said.
Assessments about required resources fall to individual business entities. Fernandes emphasised the importance of agility in choosing the right data strategies. He advised companies to stay agile in the face of failure, and switch to a new strategy to turn things around.
Bringing scale and agility to data infrastructure
Venkata Rao, of Needl.ai, shared that the challenge of building an app that scales is how companies manage their data layers. He advised companies to choose data strategies with care when they scale up. For instance, companies that use Open Search on AWS need to be sure of Elastic Block Storage (EBS) volumes and the amount of data consumed to avoid an increase in costs. Scaling of the app is dependent on how companies are able to scale their data layers, he said.
Panellists agreed that scaling horizontally helped them cope with growing demands and data. However, they cautioned companies to keep an eye on data infrastructure to ensure that scalability and uptime are maintained and discussed the cost of GPUs.
Prakash Jothirmalingam, of LeadSquared, said scaling is a continuous process. It is a journey, and companies’ expectations of products may change as they take this journey. In the initial phase, companies may strive to build their target base but once that is in place, the expectation shifts to where costs must be lowered while maintaining the same level of performance.
“Scale makes people humble. Whatever you know about architecture or engineering, the moment you start scaling, you realise that there is still something more to be done or learnt. I think it's a journey and you can continue to evolve,” Jothirmalingam said.