Data deluge: the ‘dark’ side of big data
Data generation and collection has become ingrained in businesses, organisations, and individuals around the world. The vast data sets being recorded and analysed on a regular basis have led to the term ‘big data’ becoming ubiquitous in recent years. But the repositories of this data aren't always structured and coherent. In fact, the amount of unknown and unused data being collected has led to the coining of a new term – ‘dark data’.
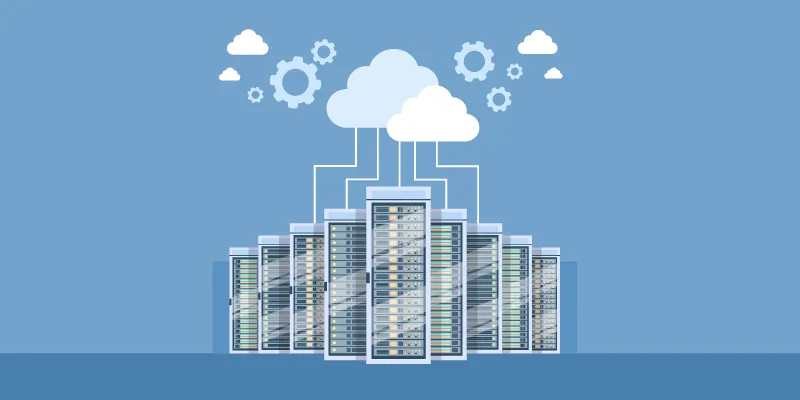
Image : shutterstock
Data is being generated at unprecedented rates today, with each new technological advancement spurring it on even further. Internet of Things, machine learning, and the digitisation of health care will soon be generating millions of gigabytes per second. Self-driving cars, too, are soon expected to enter this realm with the generation of 350 MB of data per second by 2020, according to an IMB study. But all this will come to nought if we don't change how we store, curate, structure, and analyse data. The same study stated that 80 percent of all data collected today is ‘dark’, that is, it's inactive and incoherent. An IDC study predicts that the digital universe will expand to 44 zettabytes (or 44 trillion gigabytes) by 2020, and this poses a few critical problems.
Storage and security
The biggest challenge is posed by the large volume of unstructured data — text, video, images, and audio that won’t fit into the columns and rows in a relational database. Obtained in the form of MS Office documents, instant messages, emails, social media posts, et al, this ‘dark data’ is not only difficult to analyse, but causes storage problems as well.
There are currently several options for storing big data, from hybrid clouds and flash storage to Intelligent Software Designed Storage (I-SDS) and cold storage archiving. And while the storage itself is relatively cheap, the costs associated with the maintenance and energy consumption of large data centres can be astronomical. Security is another concern associated with bid data — whether it's stored on the cloud or on local infrastructure. With data being collected from multiple sources and distributed computing being commonplace in data analysis, there are multiple avenues open for data breaches. And since it is big data that we're talking about, a breach in security will result in the compromise of a large amount of information.
Quality vs quantity
There is a dire need for organisations to focus on quality over quantity when it comes to big data. Generally speaking, the larger a data set is, the lower its quality will be. Cleaning up data, then, will involve more work than its analysis. This effort can be reduced by collecting only meaningful data. Organisations should strive to collect only that high-quality data, from both internal and external sources, which serves a purpose. But this attempt to reduce the collection of ‘dark data’ isn't always possible, in which case data exploration becomes an important preceding step.
Data exploration is the process of determining the quality of a data set and ‘efficiently extracting knowledge from data even if we do not know exactly what we are looking for’. In big data analysis, the smallest of errors can spark of subsequent miscalculations that render the entire analysis useless. Using data exploration, analysts can identify any errors that may exist before the expensive and time-consuming steps of cleaning and curating are carried out.
Big data analysis will surely undergo several changes in the coming years, if not months. Cognitive computing is already poised to leverage artificial intelligence to mine immense data sets in short spans with almost zero errors. Despite that, however, the need to reduce and streamline the big data being collected remains more important than ever.